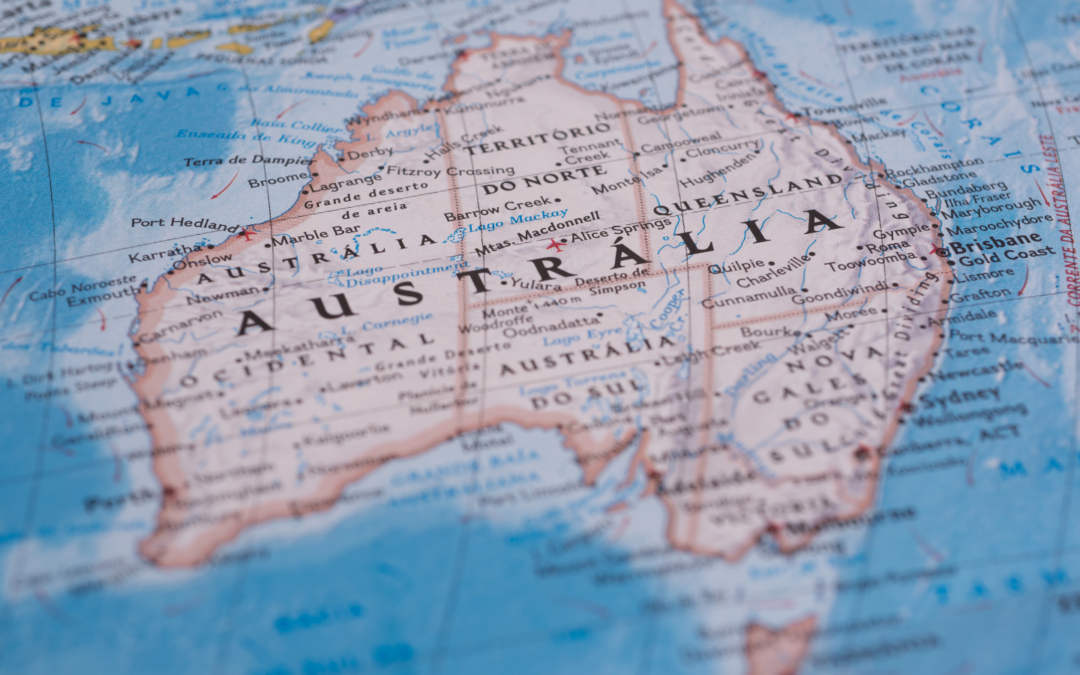
What’s the Difference Between GDA94 and GDA2020?
Geodetic datums, or geodetic systems, are often used by proptechs for mapping or analysing spatial data.
Here is a rundown of everything you need to know about the different geodetic datums we use and reference in Australia.
Table of Contents
Jump ahead ↓
What is a Geodetic Datum?
A geodetic datum is a reference framework used to define the Earth’s shape and orientation, providing a coordinate system that allows for accurate mapping, surveying, and pinpointing exact locations on the Earth’s surface.
In Australia, we use Geodetic Datum of Australia 1994 (DA94) and Geodetic Datum of Australia 2020 (GDA2020).
The History of Australia’s Geodetic Datums
Prior to GDA94, Australian surveyors primarily used the Australian Geodetic Datum 1966 (AGD66), which was based on a network of ground-based survey points and astronomical observations.
AGD66 was the standard datum used for mapping and surveying in Australia for several decades until it was superseded by GDA94 in the 1990s.
The decision to switch to GDA94 was driven by the need for a more accurate and up-to-date geodetic datum that could take advantage of advances in geospatial technology such as GPS. AGD66 was also affected by tectonic movements and other changes in the Earth’s surface, which made it increasingly difficult to use for accurate positioning and navigation.
GDA94 (Geocentric Datum of Australia 1994) was the geodetic datum used in Australia from 1994. Based on a mathematical model of the Earth’s surface defined using measurements from a network of ground-based survey points, and used as the standard datum for mapping and surveying in Australia.
Now, GDA2020 (Geocentric Datum of Australia 2020) is the current geodetic datum used in Australia. It was introduced in 2017 to replace GDA94 and is based on more recent measurements of the Earth’s surface using advanced satellite and ground-based technology.
GDA2020 provides a more accurate representation of the Earth’s surface than GDA94, and is designed to be compatible with global positioning systems (GPS) and other modern geospatial technologies.
Even though AGD66, and to some extent GDA94, are no longer the primary datums used in Australia, it’s still important to maintain historical data that was referenced to this datum. And it is possible to transform data from AGD66 to GDA94 or GDA2020 using appropriate transformation parameters to ensure compatibility and accuracy when comparing or integrating data from different sources.
Conversions between Geodetic Datums
Conversions between AGD66 and GDA94 are not 100% accurate, because the two datums are based on different mathematical models of the Earth’s surface with different reference points and parameters.
To convert data from AGD66 to GDA94 (or vice versa), a mathematical transformation must be applied that takes the differences between the two datums into account.
This transformation involves adjusting the latitude, longitude and height values of the data to align with the new datum.
However, there are many factors that can affect the accuracy of this transformation, such as:
- The quality and accuracy of the original data: If the original data was collected using imprecise or inaccurate methods, the transformation may introduce additional errors or inaccuracies.
- The complexity of the transformation: Some transformations may require more complex mathematical models or additional parameters to be specified, which can increase the likelihood of errors.
- The location and terrain of the data: The accuracy of the transformation can vary depending on the location and terrain of the data. Some areas may be more affected by tectonic movements or other changes in the Earth’s surface, which can make the transformation more challenging.
- The type of data being transformed: Different types of data (e.g. points, lines, polygons) may require different transformation methods or parameters, which can affect the accuracy of the transformation.
While conversions between AGD66 and GDA94 can be relatively precise, they’re not 100% accurate.
This is due to the inherent differences between the two datums, and the potential for errors or inaccuracies in the transformation process. It’s important to use appropriate transformation methods and understand the limitations and potential sources of error when converting data between different geodetic datums.
The Difference Between GDA94 and GDA2020
The key differences
The main difference between GDA94 and GDA2020 is their accuracy and the methods used to define them.
GDA2020 is a more accurate and up-to-date datum, with improvements in the modeling of the Earth’s surface that take into account changes in its shape over time. This means that positions and distances measured using GDA2020 are more accurate than those measured using GDA94. Additionally, GDA2020 is designed to be compatible with modern geospatial technologies and is expected to be used for many years to come.
It’s worth noting that the difference between GDA94 and GDA2020 may not be significant for many applications, particularly those that don’t require high levels of accuracy. However, for applications that require precise positioning or measurement, such as surveying or mapping, selecting the correct geodetic datum is important to ensure accurate results.
Differences in distance and direction
The average distance and direction difference between GDA94 and GDA2020 depends on the location on the Earth’s surface.
In general, the differences between the two datums are greatest in areas with high tectonic activity or areas where the Earth’s surface is undergoing significant changes, such as due to land subsidence or sea level rise.
According to Geoscience Australia, the organisation responsible for geodetic information and services in Australia, the average difference between GDA94 and GDA2020 in Australia is around 1.5 meters. However, this value can vary significantly depending on the location, with some areas showing differences of several meters or more.
The direction of the difference between the two datums also varies depending on the location, as it is related to the direction and magnitude of any tectonic movements or changes in the Earth’s surface. In general, the direction of the difference is determined by the vector between the two datums at a given location.
It’s important to note that the difference between GDA94 and GDA2020 is not constant over time and may continue to change in the future. This is because the Earth’s surface is constantly changing due to tectonic activity, sea level rise, and other factors. As such, it’s important to regularly update geodetic data and use the most up-to-date geodetic datum for accurate positioning and navigation.
Migrating from GDA94 to GDA2020
The differences between the two means that migrating from GDA94 to GDA2020 can present several challenges and issues, particularly for organisations or projects that rely heavily on geospatial data.
Some of the key issues with migrating to GDA2020 include:
- Data compatibility: Data that was created using GDA94 may not be compatible with GDA2020. This can cause issues when trying to integrate or compare datasets that use different datums.
- Application compatibility: Applications that were designed to work with GDA94 may not be compatible with GDA2020. This can require updates or modifications to existing software or the adoption of new tools.
- Training and expertise: Staff who work with geospatial data may need to be trained on the new GDA2020 datum and its associated tools and workflows. This can take time and resources.
- Time and cost: Migrating to GDA2020 can be a complex and time-consuming process, particularly for large organisations or projects. There may be costs associated with updating software, purchasing new equipment, or retraining staff.
- Accuracy: While GDA2020 is a more accurate datum than GDA94, some existing data may still be more accurate when referenced to GDA94. This can make it difficult to compare or integrate data from different sources.
- Data transformation: In some cases, it may be necessary to transform data from GDA94 to GDA2020, which can introduce errors or inaccuracies. The accuracy of the transformation depends on the quality of the original data and the transformation method used.
Migrating from GDA94 to GDA2020 requires careful planning and consideration of the potential issues and challenges. It’s crucial to work closely with geospatial experts and stakeholders to ensure a smooth and successful transition.
What is WGS84 and Why is it Used by Software?
WGS84 (World Geodetic System 1984) is a geodetic datum used for positioning and navigation purposes. It defines a reference system for the Earth’s surface that allows locations to be specified in latitude and longitude coordinates.
The WGS84 datum was developed by the United States Department of Defense for use by the military and intelligence agencies, but it has since become the standard geodetic datum used by many organisations and applications around the world, including GPS (Global Positioning System) devices and mapping software.
The WGS84 datum is based on a mathematical model of the Earth’s surface that takes into account its shape, size, and rotation. It defines a set of reference points and parameters that allow positions on the Earth’s surface to be accurately calculated and communicated.
The WGS84 datum is widely used because it is compatible with GPS and other global navigation systems, allowing precise positioning and navigation in real-time. However, while there may be regional differences in the Earth’s surface that are not fully captured by the WGS84 model, that other geodetic datums may be more appropriate for certain applications or regions.
How to Convert Between GDA2020 and WGS84
To convert between GDA2020 (Geocentric Datum of Australia 2020) and WGS84 (World Geodetic System 1984), you can use coordinate transformation parameters provided by geodetic authorities. The transformation process involves converting coordinates from one datum to another using a mathematical model.
In the case of GDA2020 and WGS84, the transformation parameters provided by the Intergovernmental Committee on Surveying and Mapping (ICSM) in Australia are known as the National Transformation Version 2 (NTv2) grid files. These grid files contain the necessary information for accurate transformations.
The accuracy of the transformation depends on the specific region and the quality of the NTv2 grid files used. Always use the most up-to-date and accurate transformation parameters available from reputable sources.
To convert coordinates between the GDA2020 (Geocentric Datum of Australia 2020) and WGS84 (World Geodetic System 1984) datums using Python, you can utilise the pyproj library. pyproj provides a convenient interface to the PROJ library, which is a widely used cartographic projection and coordinate transformation library.
Geodetic Datum Usage in Australia
In Australia, a lot of data providers offer data sets in both GDA94 and GDA2020 geodetic datums because the uptake of GDA2020 is not universal. It’s common practice for these providers to specify which datum was used to create each dataset.
When combining geospatial datasets, it’s important for data professionals to ensure consistency in the geodetic datums employed.
Using different datums without proper alignment can lead to inaccuracies, such as misaligning spatial features. For this reason, careful attention to datum consistency is essential to maintain the integrity and accuracy of integrated geospatial data.
Originally published: 5 August, 2023
Last updated: 11 February, 2025