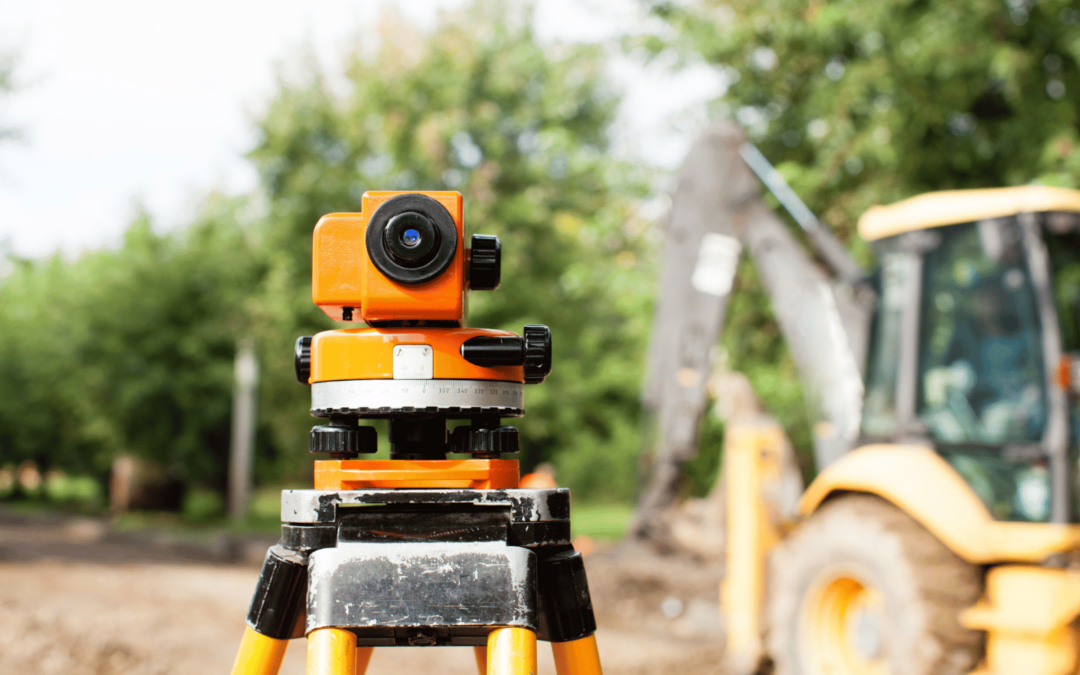
What is a Geodetic Datum?
A geodetic datum can be described as a reference point or starting line that helps us measure and describe locations on the Earth’s surface.
Let’s use a sporting example to explain the concept.
Say you’re playing baseball, and have a home base where you start your game. This home base is like a geodetic datum. It’s a fixed point on the field that everyone agrees on as the reference point for scoring runs.
In baseball, all the distances are measured from home base. For example, how far you hit the ball or how far you run around the bases is based on your relationship to that central point. Without a fixed home base, it would be challenging to keep track of scores and positions accurately.
In the world of geography and mapping, Earth’s surface is vast and not perfectly flat, so we need a similar reference point. And a geodetic datum does just this, serving as a foundation for mapping and navigation.
What is a geodetic datum?
A geodetic datum is a model that defines the shape and size of the Earth’s surface, as well as the location of a reference point (the origin) and the orientation of the axes.
A geodetic datum provides the framework for measuring and comparing positions on the Earth’s surface.
Components
A geodetic datum consists of several key components:
- Reference Ellipsoid: Describes the shape of the Earth (e.g., WGS 84).
- Geodetic Center: Specifies the Earth’s centre point.
- Prime Meridian: Defines the longitudinal reference line (e.g., Greenwich Meridian).
What purposes does a geodetic datum serve?
Geodetic datums serve as a reference framework for defining the shape of the Earth and its orientation. They provide a consistent basis for measuring latitude, longitude, elevations and are essential in geospatial analysis.
Geodetic datums help us create accurate maps, GPS navigation and positioning, and other location-based systems by giving us a standardised starting point to measure distances and positions from.
Different types of geodetic datums
Some geodetic datums are global, aiming to provide a worldwide reference framework, although many countries and regions around the world commonly use different geodetic datums to best fit the curvature of the Earth in their boundaries for greater accuracy. They may also vary from one region to another due to historical, technical, and practical reasons.
Examples of commonly used geodetic datums include the World Geodetic System 1984 (WGS84) and the North American Datum 1983 (NAD83).
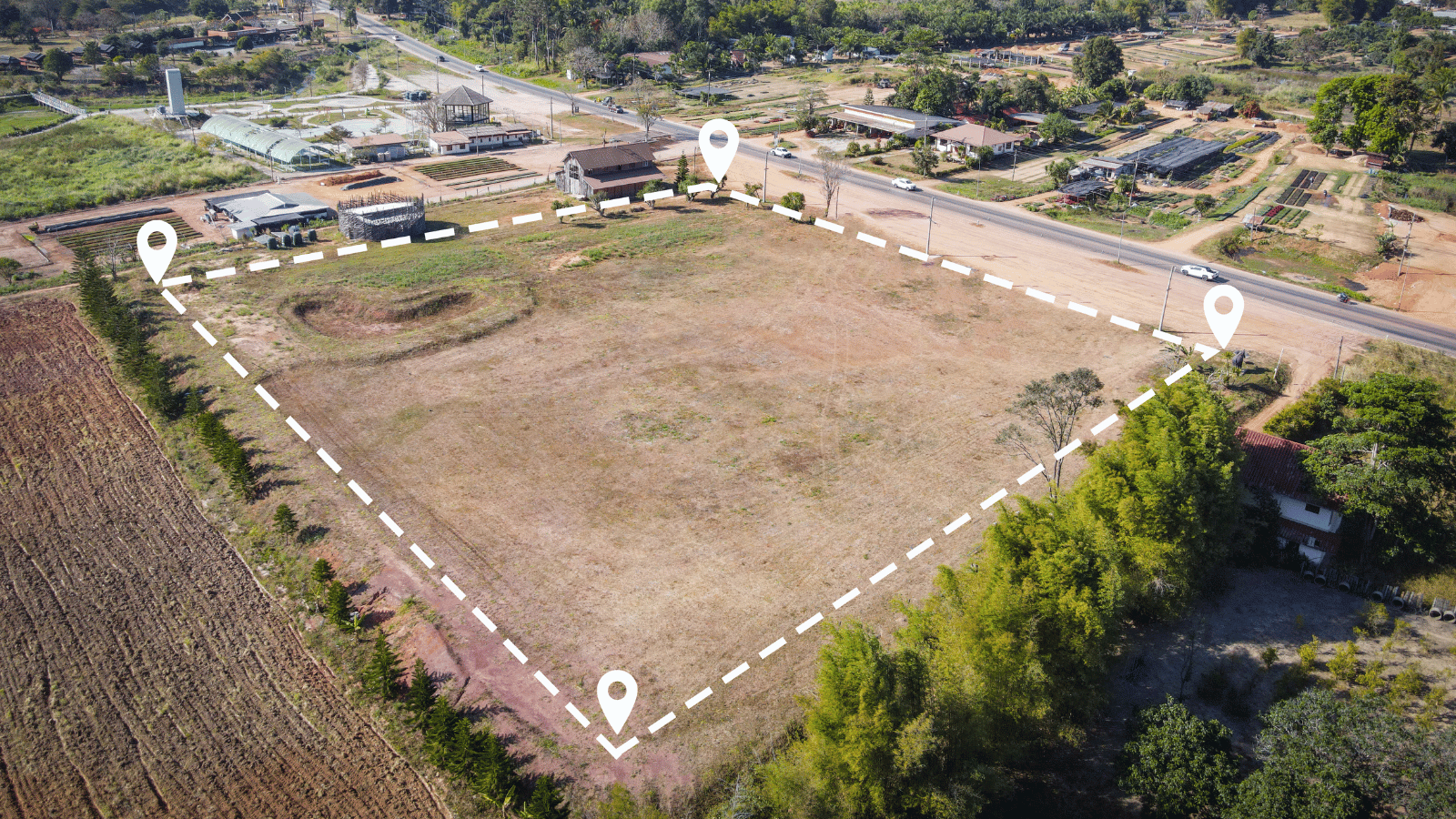
How geodetic datums are used in property and real estate
Geodetic datums are fundamental to property and real estate by providing a standard referencing system for defining property boundaries, mapping, location-based services, and decision-making processes related to land use and property transactions.
- Property Surveys:
When a property is surveyed, geodetic datums provide a reference framework for precisely locating property boundaries and corners. Surveyors use coordinates based on the datum to define the property’s position on the Earth’s surface accurately. This is critical for property boundaries, ensuring that the land’s legal description is accurate. - Land Records and Title Deeds:
Property records and title deeds often include coordinate information based on a specific geodetic datum. This information ensures the accuracy and consistency of land ownership records. - Geographic Information Systems (GIS) Mapping: GIS used in the real estate industry rely on geodetic datums to create digital maps. These maps help real estate professionals and government agencies manage property information, zoning, and land use more effectively.
- Location-Based Services:
Real estate agents and online platforms use mapping applications that rely on geodetic datums to display property locations accurately. This helps potential buyers or renters understand the property’s precise location and nearby amenities. - Property Valuation:
Geodetic datums can be used in property valuation models to consider factors like the property’s location, proximity to schools, transportation, and other geographic features. These factors can affect a property’s value. - Land Use Planning:
Urban and regional planners use geodetic datums to assess the suitability of land for different purposes, such as residential, commercial, or industrial development. They consider geographic factors and zoning regulations. - Environmental Impact Assessment:
When evaluating the environmental impact of a real estate development, geodetic datums help assess factors like proximity to water bodies, floodplains, and protected areas. - Infrastructure Development:
Geodetic datums are essential for planning and constructing infrastructure, such as roads, utilities, and public transportation systems. Accurate location information ensures that developments are built correctly. - Property Insurance:
Insurers may use geodetic datums to assess the risk associated with a property’s location, particularly regarding natural disasters like floods or earthquakes.
Geodetic datums are used widely by proptechs and serve a number of purposes in real estate and property management.
Read our blog to learn about the geodetic datums we use and reference in Australia.