AUSTRALIA
Consumer Demographics
From geoTribes
Consumer Demographics is a synthetic reconstruction of the 2021 Australian Census that’s optimized for database profiling
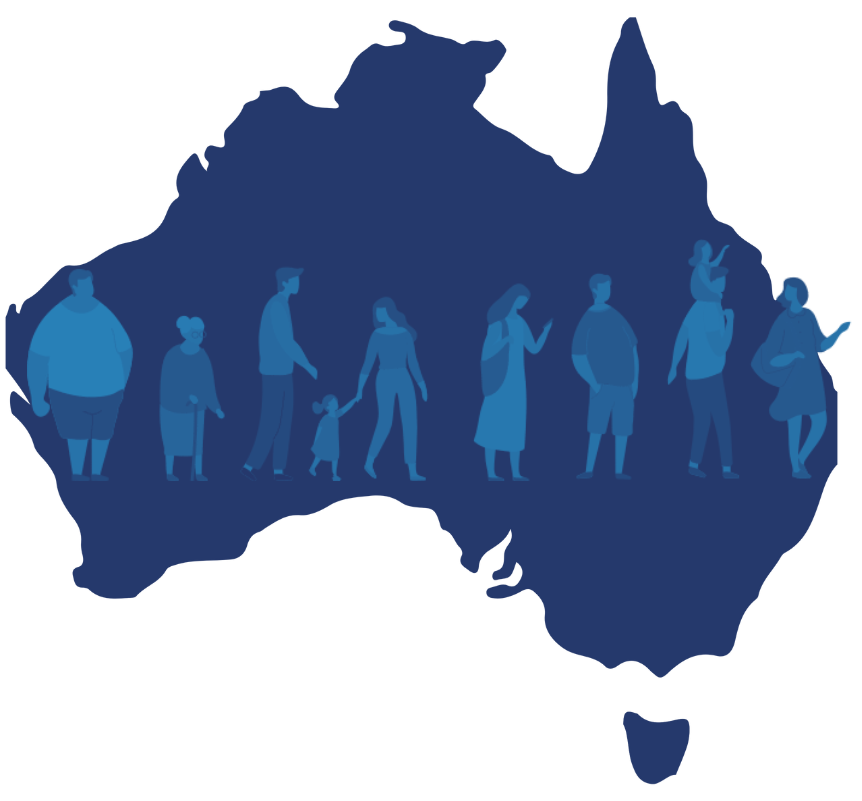
Data Overview
geoTribes Consumer Demographics is a synthetic reconstruction of the 2021 Australian Census that’s optimized for database profiling. What makes our Consumer Demographics special is its high level of match resolution.
Instead of matching databases at SA1-level with only 60K levels, our match-key of SA1 + Dwelling Type + Age Band + Gender matches at 50 times the precision in a secure, compliant, virtual and instantaneous manner with over 3.1M match levels.
This means that within a particular SA1, people in different age and gender cohorts will have different financial, cultural and life stage profiles. This higher level of accuracy really pays off in:
- Cluster Analysis,
- Predictive analytics and
- Benchmarking database cultural composition against the relevant population
This unique capability, based on our Synthetic Population Models, means that the demographic profiles on customer databases are much more accurate and responsive to an individual customer’s age and gender rather than just the SA1 they live in, leading to improved business analytics and better training of machine learning (ML) models for data science.
A trial product is provided at no charge for a 90-day evaluation.
The trial dataset includes data for the SA4 (Statistical Area Level 4) of 125 – Sydney – Parramatta.
A data dictionary for the geoTribes: Consumer Demographics can be found at https://geotribes.com/consumer-data
The data is built in a sophisticated process based on Synthetic population models that accurately represent the total household and person populations of each country, with many millions of georeferenced individual synthetic records and deep sociodemographic profiling.
Expected Workflow:
- User requests this data from geoTribes (via the Request button);
- geoTribes and user agree to commercial terms;
- geoTribes provides user with details of the share via UDTF;
- User appends geoTribes Consumer Demographics to their database through the Snowflake share;
- geoTribes Consumer Demographics available on user database for analytics, visualization and other use cases.
geoTribes: Consumer Demographics (AUS) are supplied as a Snowflake UDTF that accepts a database with the following schema as input:
– RECORDID (unique record ID)
– MATCHKEY (Either 11-character 2021 SA1 code or 4-character postcode)
– ADDTYPE (2-level coding of dwelling type)
– AGEBRK (13-level coding of age in 5-year bands)
– GENDER (2-level coding of gender)
The UDTF will return the following as additional fields for each RECORDID (expressed as probabilities to 3 decimal points):
– Household Demographics – 39 Variables
– Person Demographics: Cultural – 49 Variables
– Person Demographics: Work and Life – 85 Variables
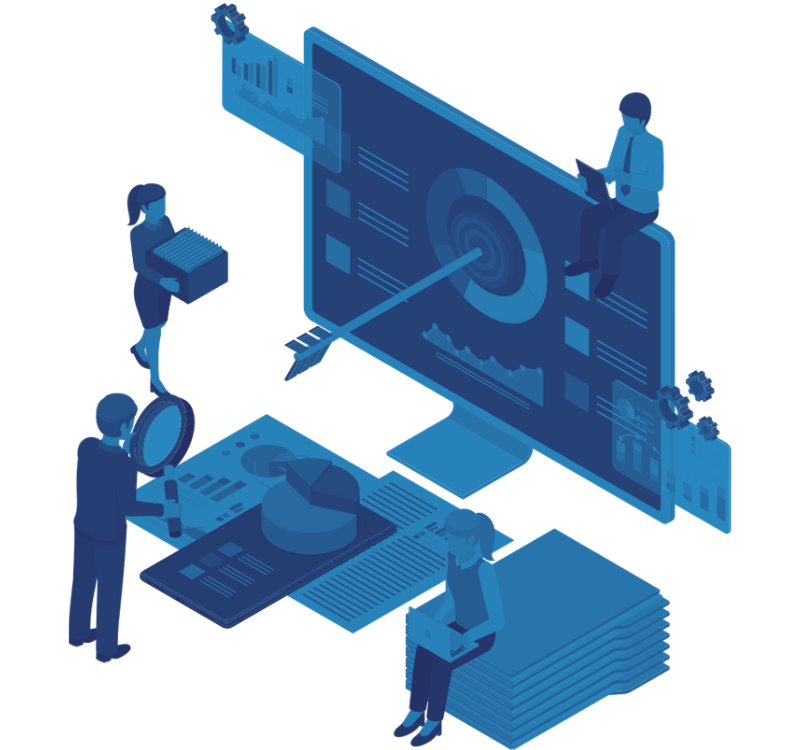